Introduction
FinTech’s embrace of Artificial Intelligence (AI), Machine Learning (ML), and Deep Learning (DL) signifies a technological leap forward in the financial sector. This blog offers a technical exploration of these technologies, focusing on neural networks and their applications in FinTech.
A Technical Exploration
FinTechs are harnessing the power of AI, ML, and DL to revolutionise various aspects of financial services. Let’s explore these technologies from a technical standpoint:
Artificial Intelligence
AI in FinTech goes beyond automating tasks; it involves creating systems that can make decisions, learn from data, and improve over time. Technologies like Natural Language Processing (NLP) enable sophisticated customer service solutions, like chatbots that understand and respond to customer queries with human-like accuracy.
American Express is leveraging AI to create more intuitive interfaces and personalised customer service experiences in every interaction. This application of AI in customer service can be a great example of how AI goes beyond simple automation to create systems that learn from data and make decisions, enhancing customer interactions in FinTech​​.
Machine Learning
ML in FinTech is about leveraging algorithms to analyse data, identify patterns, and make predictions. For instance:
- Predictive Analytics: Used in personal finance applications, ML algorithms analyse spending patterns to offer personalised financial advice.
- Risk Management: ML models assess loan applications by evaluating risk factors more comprehensively than traditional methods.
Credit Karma employs predictive credit modelling, an application of ML, to generate advisory predictions for its user base from thousands of data points. This case study illustrates how ML algorithms are used in personal finance applications to analyse data patterns and offer personalised financial services.
Deep Learning
Deep Learning, a subset of ML, utilises layered neural networks to process data in complex ways. Here’s how it’s applied in FinTech:
- Convolutional Neural Networks (CNNs): Used in fraud detection, CNNs can analyse transaction images or patterns to detect anomalies.
- Recurrent Neural Networks (RNNs): Ideal for time-series data like stock prices, RNNs can predict future trends based on historical data.
- Generative Adversarial Networks (GANs): Used in algorithmic trading, GANs involve two neural networks competing against each other to generate increasingly sophisticated trading strategies.
Stripe Radar uses a deep neural network to assess transactions for fraud detection. It analyses over 1,000 characteristics of a potential transaction to determine its legitimacy, showcasing how DL can process complex data for practical applications like fraud detection in FinTech​​.
FinTech Applications of AI, ML, and DL
These technologies are not just theoretical; their practical applications in FinTech are diverse:
- Customer Service Automation: AI-driven chatbots using NLP for customer interaction, improving service efficiency and customer satisfaction.
- Fraud Detection and Prevention: Leveraging CNNs to analyse transaction data, reducing fraudulent activities.
- Credit Scoring: Utilising RNNs to analyse creditworthiness, offering a more nuanced understanding than traditional scoring methods.
- Algorithmic Trading: GANs creating advanced trading algorithms that adapt to market changes faster than traditional models.
Cyber Security Considerations
FinTech’s integration of AI, ML, and DL technologies is transformative, but it also introduces specific cyber security challenges that require technical acumen to navigate:
AI-Driven Customer Service Security:
- NLP and Chatbots: Securing NLP-driven chatbots in FinTech involves implementing vigorous data sanitisation to prevent injection attacks, employing contextual anomaly detection to flag unusual user interactions, and securing API endpoints against unauthorised access. Additionally, regular retraining of NLP models and periodic security audits are crucial to adapt to evolving threats and ensure the integrity of these interactive systems.
ML in Financial Analysis – Protecting Data and Models:
- Predictive Analytics and Risk Management: Effective security in ML-driven predictive analytics involves implementing tailored intrusion detection systems (IDS) to monitor unusual data patterns and potential model tampering. It’s crucial to question whether your data is continuously monitored and protected at every stage. Incorporating regular red team exercises can further help in identifying and addressing vulnerabilities in your ML workflows.
Deep Learning Networks – Ensuring Integrity and Confidentiality:
- CNNs for Fraud Detection: Network segmentation and zero-trust architectures in CNNs enhance data security. Consider micro-segmentation to control network traffic and isolate parts of the system. How effective are your intrusion detection systems, especially those leveraging AI, in adapting to evolving fraud techniques?
- RNNs for Market Analysis: Combining secure multi-party computation (SMPC) with differential privacy techniques in RNNs can add a layer of security. Think about the potential benefits of federated learning models where data analysis is decentralised – could this reduce your data concentration risks?
- GANs for Algorithmic Trading: Beyond advanced threat modelling, continuous security monitoring and anomaly detection are key in algorithmic trading. Reflect on your controls for detecting unusual trading activities and consider whether your threat model is being updated regularly to incorporate the latest attack vectors and system vulnerabilities.
Reflecting on Your Cyber Security Strategy
Incorporating AI, ML, and DL into FinTech demands a rigorous approach to cyber security. Consider these critical aspects:
- Risk Management in AI Systems: How effectively are you identifying and mitigating risks specific to your AI-driven platforms? Consider aspects like data integrity, model vulnerability, and attack surfaces unique to AI applications.
- Security Controls Adaptation: Are your security controls dynamically aligned with the latest AI advancements and threat landscapes? This includes reviewing not just the frequency of updates but also the depth and relevance of these changes.
- Resilience of AI Infrastructure: How resilient is your AI infrastructure against sophisticated cyber attacks? Evaluate the robustness of your defences in the face of potential AI-specific attacks like adversarial attacks, data poisoning, and model exploitation.
These questions aim to encourage a deeper assessment of your AI cyber security posture, aligning it with the innovative pace of your FinTech operations.
Conclusion
The rapid integration of AI, ML, and DL technologies in all industries is not only reshaping the technical landscape, but also introducing a new threat landscape. As these intelligent systems become more intricate, the security frameworks that protect them must also evolve. It’s crucial to not only anticipate potential cyber threats, but also to implement resilient security measures that can adapt to the advanced capabilities of AI-powered technologies.
The cyber security elements of these technologies in financial services demands not only technical expertise, but also a proactive approach to security. At Falx, we bring a bespoke approach to securing your technological innovations. Our expertise lies in producing tailored security strategies that are as dynamic and intelligent as the AI systems they protect.
Reach out to us for a discussion, see how we can help you, and let’s enhance the cyber resilience of your applications.
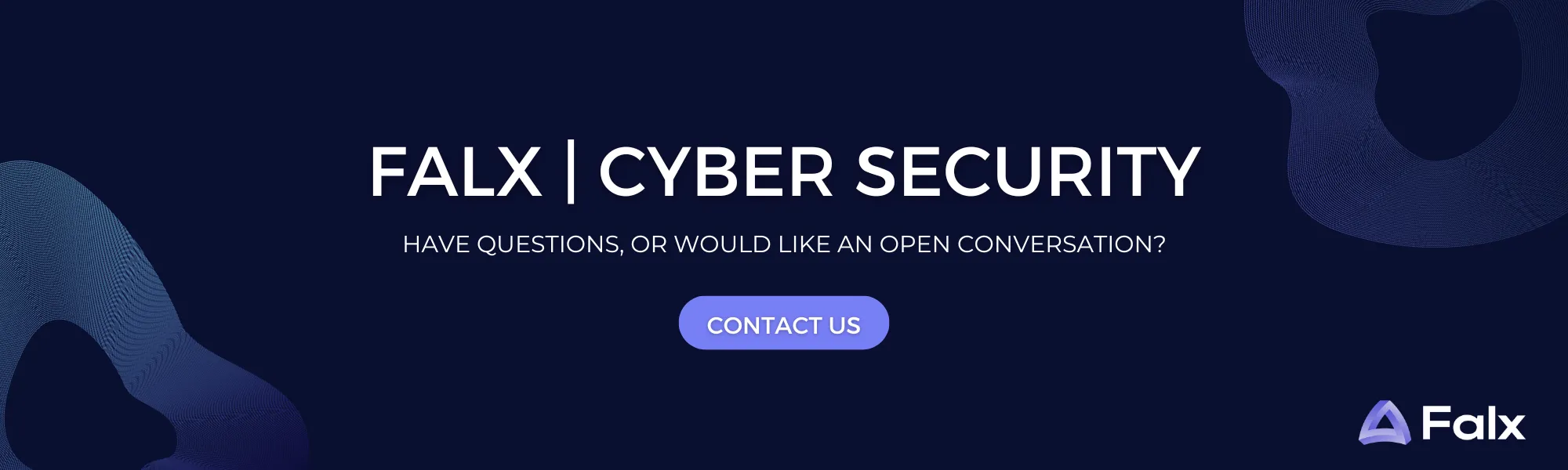